Note
Go to the end to download the full example code.
Geomodeling benchmark: the “Hecho”-Model¶
This model is part of a geomodeling benchmaring effort. More information (and, hopefully, publication) coming.
import os
import numpy as np
# Aux imports
import pandas as pn
# Importing gempy
import gempy as gp
import gempy_viewer as gpv
Loading surface points from repository:¶
With pandas we can do it directly from the web and with the right args we can directly tidy the data in gempy style:
data_path = os.path.abspath('../../data/input_data/Hecho')
dfs = []
# First stratigraphic data
for letter in range(1, 10):
dfs.append(pn.read_csv(
filepath_or_buffer=data_path + '/H' + str(letter) + '.csv',
sep=';',
names=['X', 'Y', 'Z', 'surface', '_'],
header=0
))
# Also faults
for f in range(1, 4):
fault_df = pn.read_csv(
filepath_or_buffer=data_path + '/F' + str(f) + 'Line.csv',
sep=';',
names=['X', 'Y', 'Z'],
header=0
)
fault_df['surface'] = 'f' + str(f)
dfs.append(fault_df)
# We put all the surfaces points together because is how gempy likes it:
surface_points = pn.concat(dfs, sort=True)
surface_points.reset_index(inplace=True, drop=False)
surface_points.tail()
Now we do the same with the orientations:
orientations = pn.read_csv(
filepath_or_buffer=data_path + '/Dips.csv',
sep=';',
names=['X', 'Y', 'Z', 'G_x', 'G_z', '_'],
header=0
)
# Orientation needs to belong to a surface. This is mainly to categorize to which series belong and to
# use the same color
orientations['surface'] = 0
# We fill the laking direction with a dummy value:
orientations['G_y'] = 0
# Replace -99999.00000 with NaN
orientations.replace(-99999.00000, np.nan, inplace=True)
# Drop irrelevant columns
orientations.drop(columns=['_'], inplace=True)
# Remove rows containing NaN
orientations.dropna(inplace=True)
Data initialization:¶
Suggested size of the axis-aligned modeling box: Origin: 0 -0.5 0 Maximum: 16 0.5 4.5
Suggested resolution: 0.05m (grid size 321 x 21 x 91)
%%
surface_points_table: gp.data.SurfacePointsTable = gp.data.SurfacePointsTable.from_arrays(
x=surface_points['X'].values,
y=surface_points['Y'].values,
z=surface_points['Z'].values,
names=surface_points['surface'].values.astype(str)
)
orientations_table: gp.data.OrientationsTable = gp.data.OrientationsTable.from_arrays(
x=orientations['X'].values,
y=orientations['Y'].values,
z=orientations['Z'].values,
G_x=orientations['G_x'].values,
G_y=orientations['G_y'].values,
G_z=orientations['G_z'].values,
names=orientations['surface'].values.astype(str),
name_id_map=surface_points_table.name_id_map # ! Make sure that ids and names are shared
)
structural_frame: gp.data.StructuralFrame = gp.data.StructuralFrame.from_data_tables(
surface_points=surface_points_table,
orientations=orientations_table
)
geo_model: gp.data.GeoModel = gp.create_geomodel(
project_name='Moureze',
extent=[0, 16, -0.5, 0.5, 0, 4.5],
resolution=[321, 21, 91],
structural_frame=structural_frame
)
gp.set_section_grid(
grid=geo_model.grid,
section_dict={
'section': ([0, 0], [16, 0], [321, 91])
},
)
Active grids: GridTypes.NONE|SECTIONS|DENSE
We need an orientation per series/fault. The faults does not have orientation so the easiest is to create an orientation from the surface points availablle:
f_names = ['f1', 'f2', 'f3']
for fn in f_names:
element = geo_model.structural_frame.get_element_by_name(fn)
new_orientations = gp.create_orientations_from_surface_points_coords(
xyz_coords=element.surface_points.xyz
)
gp.add_orientations(
geo_model=geo_model,
x=new_orientations.data['X'],
y=new_orientations.data['Y'],
z=new_orientations.data['Z'],
pole_vector=new_orientations.grads,
elements_names=fn
)
Now we can see how the data looks so far:
gpv.plot_2d(geo_model)
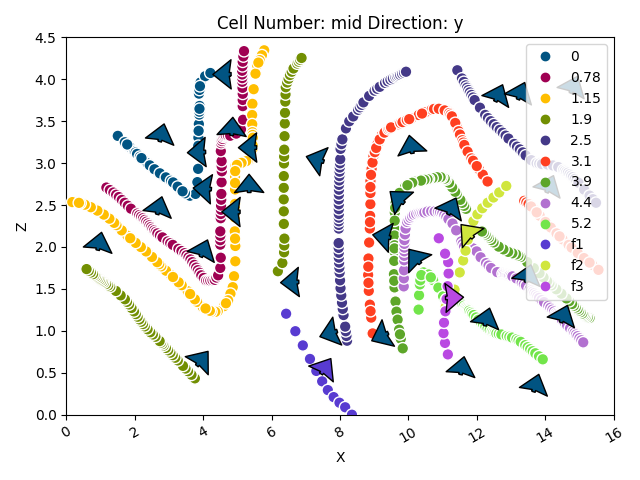
<gempy_viewer.modules.plot_2d.visualization_2d.Plot2D object at 0x7fbc9e9f4f10>
By default all surfaces belong to one unique series.
We will need to separate with surface belong to each series:
gp.map_stack_to_surfaces(
gempy_model=geo_model,
mapping_object={'Fault1': 'f1', 'Fault2': 'f2', 'Fault3': 'f3'}
)
However if we want the faults to offset the “Default series”, they will need to be more recent (higher on the pile). We can modify the order by:
Lastly, so far we did not specify which series/faults are actula faults:
gp.set_is_fault(
frame=geo_model,
fault_groups=['Fault1', 'Fault2', 'Fault3']
)
The default range is always the diagonal of the extent. Since in this model data is very close we will need to reduce the range to 5-10% of that value:
geo_model.interpolation_options.kernel_options.range *= 0.2
Explanation of model characteristics and adjustments This model has characteristics that make it difficult to get the right default values: - It is large, and we want high resolution - Some series have a large conditional number (i.e., the model input is not very stable) To address these issues: - Reduce the chunk size during evaluation to trade speed for memory - Reduce the std of the error parameter in octree refinement, which evaluates fewer voxels but may leave some without refinement Enable debugging options to help tune these parameters.
Setting verbose and condition number options for debugging
geo_model.interpolation_options.kernel_options.compute_condition_number = True
Observations and parameter adjustments The octree refinement is making the octree grid almost dense, and smaller chunks are needed to avoid running out of memory. Adjusting parameters accordingly:
geo_model.interpolation_options.evaluation_options.evaluation_chunk_size = 50_000
gp.compute_model(
gempy_model=geo_model,
engine_config=gp.data.GemPyEngineConfig(
backend=gp.data.AvailableBackends.PYTORCH,
dtype='float64'
)
)
Setting Backend To: AvailableBackends.PYTORCH
Condition number: 1546449.733468359.
Chunking done: 206 chunks
Condition number: 1545102.643054532.
Chunking done: 258 chunks
Condition number: 1447785.0114925415.
Chunking done: 206 chunks
Condition number: 40165630.352466166.
Chunking done: 10548 chunks
Chunking done: 14 chunks
Chunking done: 14 chunks
Chunking done: 21 chunks
Chunking done: 21 chunks
Chunking done: 80 chunks
Chunking done: 80 chunks
Chunking done: 11 chunks
Chunking done: 14 chunks
Chunking done: 11 chunks
Chunking done: 550 chunks
Chunking done: 38 chunks
Chunking done: 35 chunks
Chunking done: 33 chunks
Chunking done: 8 chunks
Chunking done: 10 chunks
Chunking done: 8 chunks
Chunking done: 389 chunks
gpv.plot_2d(geo_model, cell_number=[10], series_n=3, show_scalar=True)
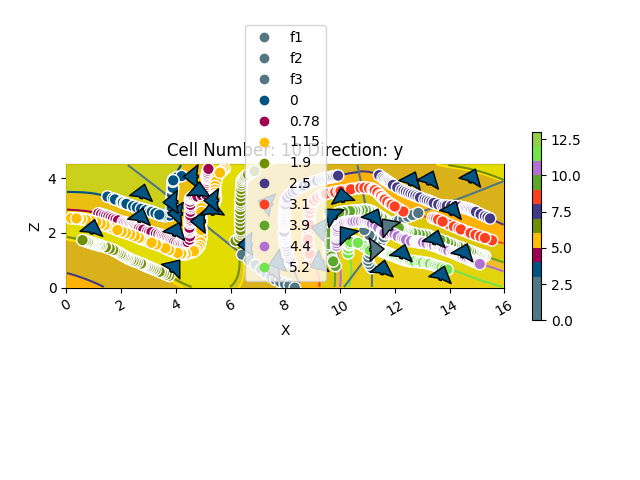
<gempy_viewer.modules.plot_2d.visualization_2d.Plot2D object at 0x7fbc9e9f48b0>
gpv.plot_2d(geo_model, cell_number=[10], show_data=True)
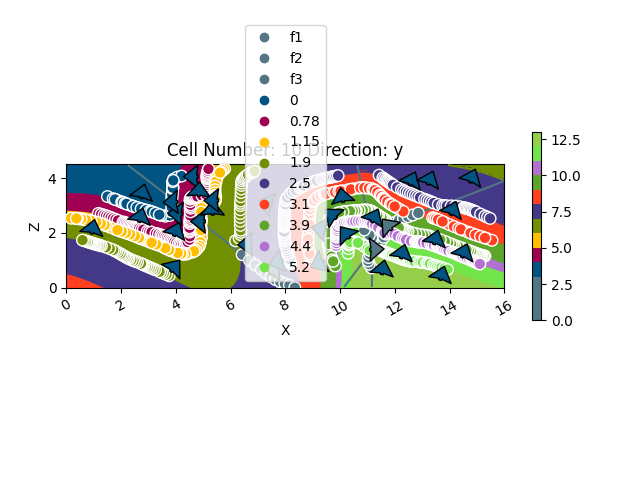
<gempy_viewer.modules.plot_2d.visualization_2d.Plot2D object at 0x7fbc9e919ae0>
gpv.plot_2d(geo_model, section_names=['section'], show_data=True)
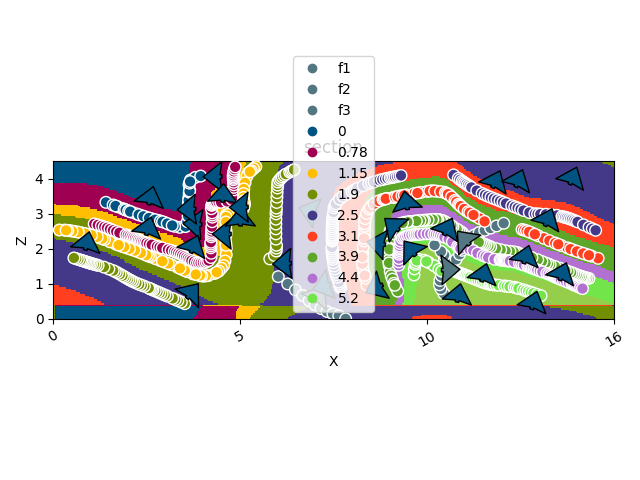
/home/leguark/TeamCity/work/3a8738c25f60c3c9/venv/lib/python3.10/site-packages/gempy_viewer/API/_plot_2d_sections_api.py:106: UserWarning: Section contacts not implemented yet. We need to pass scalar field for the sections grid
warnings.warn(
<gempy_viewer.modules.plot_2d.visualization_2d.Plot2D object at 0x7fbcb69b1600>
sphinx_gallery_thumbnail_number = 3
gpv.plot_3d(geo_model, kwargs_plot_structured_grid={'opacity': .2})
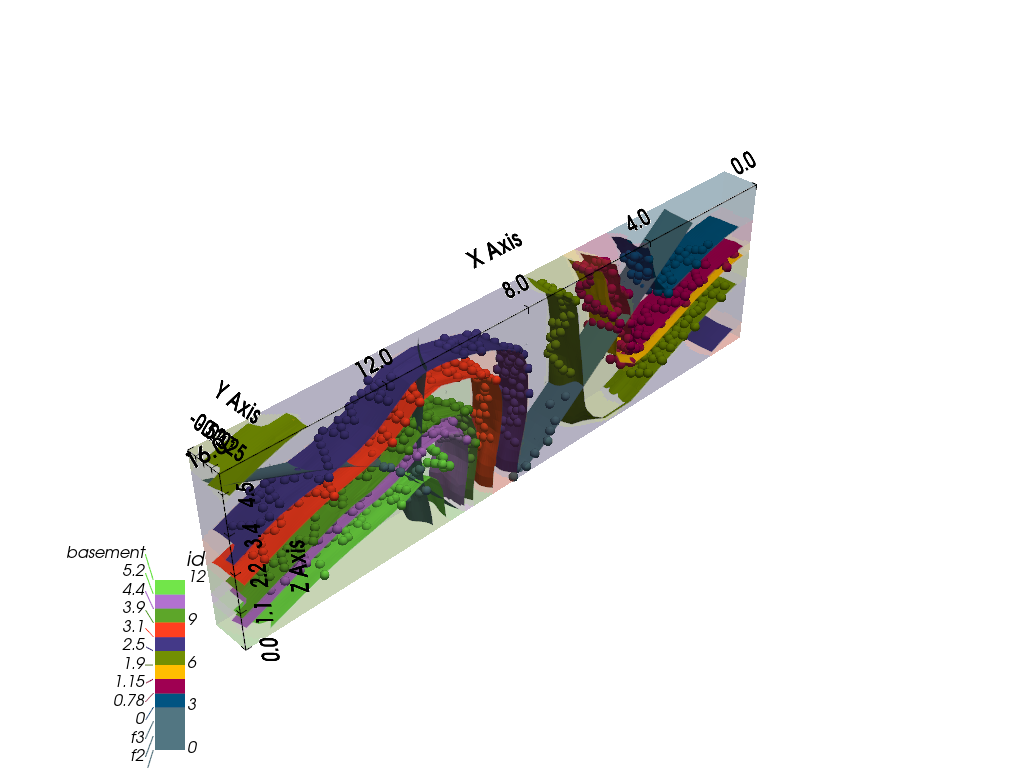
<gempy_viewer.modules.plot_3d.vista.GemPyToVista object at 0x7fbc9eb33310>
Total running time of the script: (1 minutes 38.634 seconds)